Data Quality and Quantity in AI: Ensuring Comprehensive and Representative Data Sets
Ronsley Vaz
4 min read
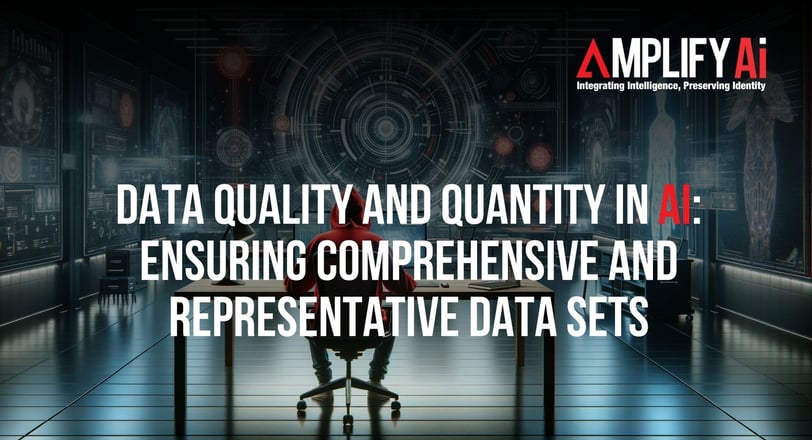
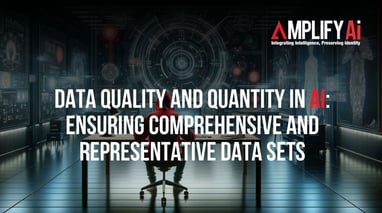
Artificial Intelligence (AI) has become integral to today's business landscape, revolutionising how organisations operate and make decisions. At the heart of AI lies data - the fuel that powers intelligent algorithms and enables machines to learn and make predictions. However, the success of AI systems heavily relies on the quality and quantity of the data they are trained on. This article will delve into the crucial topic of data quality and quantity in AI and how businesses can ensure comprehensive and representative data sets to drive ethical and effective AI implementation.
To gain deeper insights on this matter, we turn to Leopold Ajami, an expert in AI and its applications in business. Ajami emphasises that comprehensive and representative data sets are paramount in AI. They serve as the foundation upon which AI models are built, influencing AI systems' accuracy, fairness, and performance. AI can perpetuate biases and produce flawed outcomes without diverse and inclusive data. It is, therefore, imperative for businesses to prioritise data quality and quantity in their AI initiatives.
The Significance of Comprehensive and Representative Data Sets in AI
To comprehend the importance of comprehensive and representative data sets in AI, let's first understand their role in training AI models. When an AI system is trained, it learns patterns and makes predictions based on the data it has been exposed to. The AI will struggle to make accurate and fair decisions if the data is incomplete or biased. For instance, consider a recruitment AI system that is trained on historical data. If the data predominantly represents a specific gender or ethnicity due to past biases, the AI may unintentionally favour those demographics, perpetuating discrimination. Therefore, to ensure fair and equitable AI applications, it is crucial to have comprehensive and representative data sets that encompass diverse perspectives and backgrounds.
Challenges in Obtaining Comprehensive and Representative Data Sets
Obtaining comprehensive and representative data sets can be a challenging endeavour for businesses. One of the primary difficulties lies in acquiring large and diverse data sets that accurately reflect the real-world scenarios the AI will encounter. Moreover, data bias is a prevalent concern. Biases can be inadvertently introduced into AI systems if the training data itself is biased or lacks diversity. This can lead to skewed outcomes and reinforce existing inequalities. For example, a facial recognition AI trained primarily on data from lighter-skinned individuals may struggle to accurately recognise darker-skinned faces, leading to biased identification or exclusion. Additionally, the ethical considerations surrounding the use of personal data and the need for privacy protection further complicate the task of obtaining comprehensive and representative data sets.
Strategies for Improving Data Quality and Quantity
While challenges exist, there are strategies businesses can employ to enhance data quality and quantity in AI. Data governance and management practices are crucial in ensuring data quality. Establishing clear guidelines and standards for data collection, storage, and usage can help mitigate biases and ensure the accuracy and reliability of the data. Additionally, data augmentation techniques can be employed to enhance data diversity. Businesses can enrich their data sets and ensure representation across various dimensions by artificially generating new data instances or modifying existing ones.
Collaboration and data sharing between organisations also play a vital role in building comprehensive data sets. Businesses can collectively create more robust and representative data sets by pooling resources and sharing anonymised data. This approach enables organisations to leverage each other's strengths and overcome data limitations individually. By working together, businesses can ensure that the constraints of individual data sets do not limit AI systems but instead benefit from a more extensive, diverse, and accurate data pool.
The Role of Human Oversight in Data Collection and Curation
While advancements in automation have enabled the collection and curation of vast amounts of data, human oversight remains crucial in ensuring data quality and addressing biases. Humans can exercise judgment and make ethical decisions that machines cannot replicate. Human involvement in data collection and curation processes allows for identifying and correcting biases, ensuring that AI systems are fair and unbiased. It is important to balance automated data collection and human oversight to avoid unintended consequences and promote ethical AI implementation.
The Future of Data Quality and Quantity in AI
As technology evolves, new approaches and technologies are emerging to improve data quality and quantity in AI. Federated learning, for example, allows AI models to be trained on decentralised data sources without data sharing, thus addressing privacy concerns. Similarly, distributed data systems enable organisations to collaborate and share data while controlling individual data sets. These advancements can potentially enhance data quality and quantity in AI while respecting privacy and data ownership.
However, it is essential to recognise that AI is dynamic and ever-evolving. Ongoing research and development are necessary to address emerging challenges and ensure the continuous improvement of data quality and quantity in AI. As new technologies and methodologies emerge, businesses must stay informed and adapt to the changing landscape to harness the full potential of AI technology ethically and effectively.
Comprehensive and representative data sets are vital for ethical and effective AI implementation. Businesses must recognise the significance of data quality and quantity in driving accurate, fair, and unbiased AI systems. Organisations can ensure the availability of diverse and inclusive data sets by prioritising data governance, collaboration, and human oversight. Furthermore, advancements in technology, such as federated learning and distributed data systems, promise to improve data quality and quantity in AI while respecting privacy concerns. Businesses must invest in enhancing data quality and quantity to unlock the full potential of AI technology and drive responsible and impactful AI applications.
Remember, AI is only as good as the data it learns from. Let's strive for data that truly represents our world and fosters fairness, inclusivity, and innovation.
Subscribe to the podcast
Subscribe now to "Amplify Ai" and let's set sail together on this exciting voyage towards business growth and success.

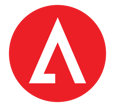
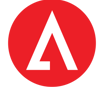
The Amplify AI Book
Amplify AI Scorecard
(c) We Are Podcast PTY. LTD. & We Are Podcast LLC. FZ.