Why you should learn machine and deep learning
Ronsley Vaz
4 min read
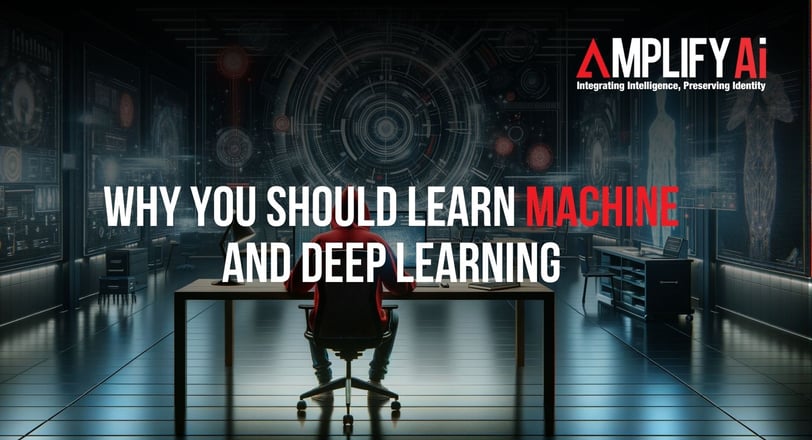
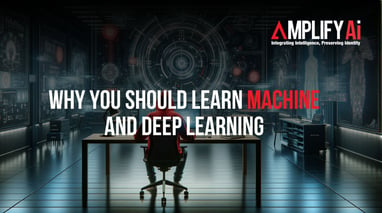
Artificial intelligence (AI) is transforming the world in once unimaginable ways. At the heart of this transformation are two concepts often used interchangeably: machine learning and deep learning. In this article, we will explore the difference between machine learning and deep learning and how they are relevant in daily technological use.
Machine learning is artificial intelligence that allows a computer to learn from data and make decisions or predictions. It works by analysing data, identifying patterns, and making predictions based on those patterns. On the other hand, deep learning is a subset of machine learning that uses neural networks to learn from large amounts of data.
Understanding these two concepts' differences is important because they have different approaches and applications. The following sections will explore the basic concepts, key components, and applications of machine learning and deep learning.
Basic Concepts
Machine learning is based on teaching a computer to recognise patterns in data. Imagine you're trying to teach a child to recognise a dog. You might show them pictures of dogs until they start to understand what a dog looks like. Machine learning does the same thing but with computers.
Deep learning takes this concept to the next level by using neural networks to learn from large amounts of data. A neural network is a set of algorithms that mimic the human brain. By using layers of artificial neurons, deep learning models can learn to recognise complex patterns in data. With thousands or millions of labelled images, the model can learn to accurately identify objects, people, or animals within a new image it has never seen before.
Key Components
The three critical components of machine learning and deep learning are data, algorithms, and neural networks.
Data is the fuel for machine learning and deep learning. The more data, the better the systems can learn. Data trains machine learning models to recognise patterns and make predictions. In deep understanding, data trains neural networks to recognise complex patterns and make more accurate predictions.
Algorithms are the instructions that tell machine learning and deep learning models how to process and learn from the data. Algorithms are like the recipe for a machine learning model. They tell the model what to look for and how to make predictions based on that data.
Neural networks are the brains of deep learning systems. They are designed to mimic how the human brain works, using layers of artificial neurons to learn from large amounts of data. This makes deep learning so powerful, as it allows for recognising complex patterns and making more accurate predictions.
Applications
Machine learning and deep learning have various applications in various industries. Here are some examples:
Recommendation systems: Recommendation systems are like personal shoppers. They learn from your past behaviour to suggest products or services you like. This is a typical use of machine learning and deep learning. For example, Netflix uses machine learning to suggest movies and TV shows based on what you have previously watched. Another example of a recommendation system is the music streaming service Spotify, which uses machine learning to suggest songs and playlists based on your listening history and preferences.
Image recognition: Image recognition is like a super-powered version of "I Spy." Deep learning systems can be trained to recognise anything from cats in photos to cancer in medical images. This is very powerful, as it detects complex patterns that would be difficult for a human to identify. Another example of image recognition is facial recognition technology, which security systems use to identify individuals.
Natural Language Processing: Natural Language Processing (NLP) is like teaching a computer to understand and speak human languages. This is a complex task that often uses both machine learning and deep learning to execute. Chatbots are becoming more popular because they can understand and respond to natural language queries. They can also analyse text to determine the writer's overall sentiment or opinion. This can be useful for businesses to gauge customer satisfaction based on reviews or social media comments.
Relevance in Daily Technological Use
Machine learning and deep learning are becoming increasingly relevant in our daily lives. They are used in popular apps and services like Netflix, Amazon, and Siri. They are transforming industries such as healthcare and finance.
Machine learning and deep learning are used in healthcare to diagnose diseases, predict patient outcomes, and develop personalised treatment plans. These technologies detect fraud, make investment predictions, and manage risk in finance.
The retail industry is also utilising machine and deep learning artificial intelligence. One example is personalised product recommendations, where machine learning algorithms analyse a customer's purchase history and browsing behaviour to suggest products they may be interested in. Deep learning is also used for image recognition in retail, allowing for automated product tagging and inventory management.
In conclusion, machine learning and deep learning are two concepts at the heart of the AI revolution. Understanding these two concepts' differences is important because they have different approaches and applications. Machine learning is about teaching a computer to learn from data and make predictions. In contrast, deep learning is a subset of machine learning that uses neural networks to learn from large amounts of data.
Machine learning and deep learning have various applications in various industries and are becoming increasingly relevant daily. As these technologies evolve, they will continue transforming the world around us.
Subscribe to the podcast
Subscribe now to "Amplify Ai" and let's set sail together on this exciting voyage towards business growth and success.

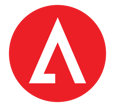
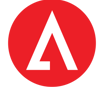
The Amplify AI Book
Amplify AI Scorecard
(c) We Are Podcast PTY. LTD. & We Are Podcast LLC. FZ.